Generative AI, or generative adversarial networks (GANs), is a groundbreaking advancement in artificial intelligence that paves the way for unparalleled creativity and innovation in various industries, including the legal world. Unlike other AI technologies, generative AI goes beyond simple data analysis and prediction; it has the ability to generate new, original content, such as images, texts, and even music. At its core, generative AI involves two neural networks, a generator and a discriminator, that work together in a competitive and iterative process to produce realistic and high-quality outputs. In this document, we will delve into the intricacies of generative AI, exploring how it works, the potential applications in the legal field, and how it differs from other advances in AI, providing lawyers with valuable insights into this cutting-edge technology.
Understanding Generative AI
Defining Generative AI
Generative AI refers to artificial intelligence systems designed to create content. This technology is based on the concept of generative adversarial networks, or GANs. Here, “generative” means the AI can produce something new, while “adversarial” and “networks” describe the interactive nature of the two neural networks involved. One network, the generator, creates content, and the other network, the discriminator, evaluates it. The discriminator’s role is to determine whether the content is distinguishable from human-created content. Through this process, the generator learns to produce increasingly sophisticated outputs. This ability to generate original, high-quality content positions generative AI as a significant leap forward from traditional AI, which primarily focuses on recognizing patterns and making predictions based on existing data.
How Generative AI Works
Generative AI operates through a system of two competing neural networks: the generator and the discriminator. The generator creates new data instances, while the discriminator evaluates them against a set of real data. These networks are trained simultaneously in a zero-sum game; as the generator improves at creating realistic outputs, the discriminator concurrently becomes better at distinguishing genuine data from fabricated data. This iterative process continues until the generator produces outputs that the discriminator can no longer easily differentiate from authentic data. The result is an AI capable of producing highly convincing, original content that mirrors the complexity and variability found in real-world data. The efficiency of this process allows generative AI to adapt and evolve, making it a powerful tool within fields that require a high degree of creativity and innovation.
Differentiating Generative AI from Other AI Advances
Unique Characteristics of Generative AI
Generative AI stands out from other AI technologies due to its unique ability to generate novel content. Unlike traditional AI, which typically analyzes and interprets existing data, generative AI can create entirely new data that mimics the statistical properties of its training set. This includes synthesizing realistic images, text, and sounds that were never previously encountered by the AI. Another distinctive feature is its self-improving mechanism. As the generative AI iterates through the process of content creation and evaluation, it refines its abilities without explicit programming for improvement. This continuous learning makes it particularly adept at tasks that require innovation and adaptation. Additionally, the outputs of generative AI are not limited by the biases or perspectives of human creators, allowing for the generation of diverse and unbiased content. These characteristics make generative AI a transformative tool in industries that value originality and efficiency.
Comparative Overview of AI Progression
AI technology has evolved significantly from its early days of rule-based systems to the complex neural networks in use today. Initially, AI was designed to perform specific, well-defined tasks, following algorithms with clear instructions. The progression led to machine learning, where AI systems learned from datasets to make predictions or categorize information, albeit within the scope of their training data. The advent of deep learning introduced neural networks capable of learning from vast amounts of data with minimal human supervision, recognizing intricate patterns and making more nuanced decisions.
Generative AI represents a further evolution of this technology. It not only recognizes patterns but also generates new data that is indistinguishable from real-world content. This ability places generative AI at the forefront of the AI field, pushing the boundaries of what machines can create and how they can be used to simulate human-like creativity and problem-solving abilities. It marks a shift from AI that understands to AI that innovates.
Generative AI’s Role in the Legal World
Potential Applications in Legal Practice
Generative AI has significant potential to revolutionize legal practice. One of its applications is in the automation of document drafting. By training on a vast array of legal documents, generative AI can produce drafts of contracts or other legal documents, saving time and reducing the risk of human error. It can also be used in predictive analysis, helping lawyers assess the likely outcomes of cases based on historical data. Another application is in the area of legal research, where generative AI can sift through law databases to find relevant precedents and statutes, streamlining the research process.
Furthermore, generative AI can assist in creating personalized legal advice by generating documents that address specific client circumstances. This technology can also be employed in simulating court proceedings or negotiations to train lawyers and law students. These applications demonstrate generative AI’s capacity to enhance efficiency and accuracy in the legal field.
Case Studies: Generative AI in Legal Workplaces
The impact of generative AI in the legal sector can be illustrated through several case studies. For instance, some law firms have implemented AI to draft and review contracts, reducing turnaround times from several hours to mere minutes. In one notable example, a legal team used generative AI to analyze and predict the outcomes of intellectual property disputes, which then informed their litigation strategy, ultimately resulting in higher success rates.
Another case involved a corporate legal department using generative AI for due diligence during mergers and acquisitions. The AI system quickly generated reports summarizing thousands of documents, highlighting potential legal issues. This not only expedited the process but also allowed lawyers to focus on higher-level strategic work. These case studies underline the tangible benefits of generative AI in legal workplaces, showcasing its potential to transform traditional legal processes.
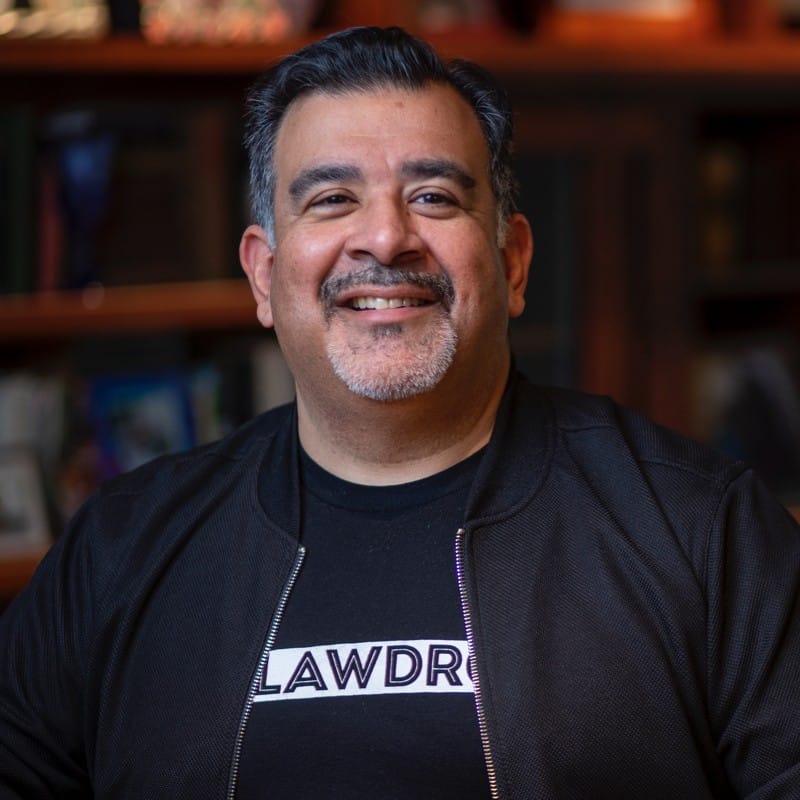
Tom Martin is a legal AI advocate, lawyer, author and speaker.
He is CEO and founder of LawDroid, a Generative AI Legal Technology company, and co-founder of the American Legal Technology Awards.
Tom has been recognized as an ABA Legal Rebel and Fastcase 50 Honoree.
Tom has presented as a speaker at ABA Techshow, LegalWeek, ILTACON, Clio Con, Legal Innovators, and many others. Tom’s writing has been published in the ABA’s Law Practice Today, Law Technology Today and GP Solo Magazine, to name a few; he has also been featured on numerous podcasts, including Legal Rebels, Un-Billable Hour, Lawyerist, the Digital Edge, and New Solo.
Tom also has his own podcast: LawDroid Manifesto on iTunes. Subscribe to his Substack at lawdroidmanifesto.com.
He serves as a mentor at the Yale Tsai Center for Innovative Thinking and ATJ Tech Fellows. Tom is a graduate of Yale and UCLA School of Law.
Born and raised in Los Angeles, California, Tom now lives in Vancouver, Canada with his wife and two daughters.